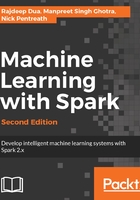
Vector spaces
Linear algebra is well known as an algebra of vector spaces. Vector objects of field type real or complex can be added and scaled by multiplying the vector with the scalar number α.
Vector space is a collection of vector objects, which can be added and multiplied together. Two vectors can be combined to produce a third vector or another object in a vector space. The axioms of vector space have useful properties. Space in vector space helps in studying the properties of physical space, for example, to find how near or far away an object is. One of the examples of vector space is a collection of vectors in a three-dimensional Euclidean space. Vector space V over field F has the following properties:
- Vector addition: denoted by v + w, where v and w are element of space V
- Scalar multiplication: denoted by α * v, where α is an element of F
- Associativity: represented by u + (v + w) = (u + v) + w, where u, v, and w are elements of space V
- Commutative: indicated by v + w = w + v
- Distributive: denoted by α * (v + w) = α * v + α * w
In machine learning, features are the dimensions of vector space.